Next: Structure among unknown variables
Up: Bayesian probability theory
Previous: Representations of data and
Contents
The Bayes rule was formulated by
reverend Thomas Bayes in the 18th century (Bayes, 1958).
It can be derived from very basic axioms (Cox, 1946).
The Bayes rule tells how to update ones beliefs when receiving new
information. In the following,
stands for the assumed model,
stands for
observation (or data), and
stands for unknown
variables.
is the prior distribution, or the
distribution of the unknown variables before making the observation. The
posterior distribution is
The term
is called the likelihood of the
unknown variables given the data and the term
is called the
evidence (or marginal likelihood) of the model.
The marginalisation principle specifies how a learning system can
predict or generalise. The probability of observing
with prior
knowledge of
is
 |
(2.2) |
It means that the probability of observing
can be acquired by
summing or integrating over all different explanations
. The term
is the probability of
given a particular
explanation
and it is weighted with the probability of the
explanation
.
Using the marginalisation principle, the evidence term can be written as
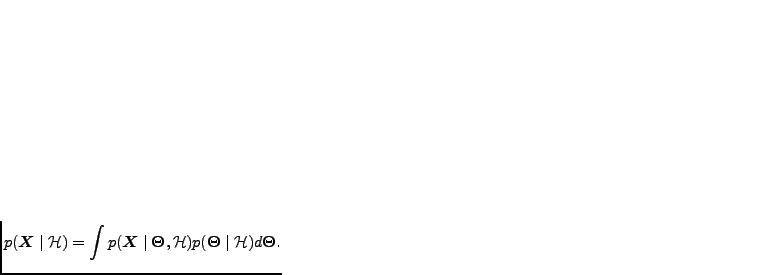 |
(2.3) |
This emphasises the role of the evidence term as a normalisation
coefficient. It is an integral over the numerator of the Bayes rule
(2.1). Sometimes it is impossible to compute the
integral exactly, but fortunately it is not always necessary. For example,
when comparing posterior probabilities of different instantiations of
hidden variables, the evidence cancels out.
Next: Structure among unknown variables
Up: Bayesian probability theory
Previous: Representations of data and
Contents
Tapani Raiko
2006-11-21