11 Control and modelling
11.1 Active sludge plant supervision and quality control
Goals
Metsä-Serla Oy, Kyro Board Mill and Neles Automation
(formerly Valmet Automation Inc.) carried out an R&D project at
Kyro Board Mill, the target of this project
was to utilize the possibilities of the modern automation system in total
control of an effluent water treatment plant.
The first experiences of applying
fuzzy logic to an effluent water treatment process were gained in 1996 at
Metsä-Serla Oy, Kyro Board Mill, in Finland. The results from Kyro indicate a
clear increase in the overall controllability of the process. The XDi control
solution based on fuzzy logic (nutrient dosage control and the return
and excess sludge removal controls) provides over 98
% availability.
Diminishing environmental load
The excess sludge control has optimized the sludge age from
12 to 7 days increasing the amount of excess sludge removal from biosludge
cycle. At the same time, there has been an increase in buffer capacity in the
biosludge thickening pool due to more efficient sludge drying.
The plant also showed a clear improvement in the suspended
solids and phosphorus reduction, as well as a remarkable 35 % decrease in the
phosphorus acid consumption.
Since fuzzy logic has been in use,
the suspended solids load has decreased from about 4.5 kg/t to below 2.0 kg/t,
and the phosphorus load from over 4 kg/d to below 2 kg/d. At the same time, the
total sludge handling capacity has increased from 36 m3/h to over 52 m3/h. The
new control solution has also helped to decrease the usage of polymer from 3.3
kg/t to 1.8 kg/t.
Methods
Efficient management of effluents requires versatile
information facilities and intelligent controls. Damatic XDi is a unique platform for integrating information management
and controls into one system. Advanced
control algorithms, such as fuzzy logic, are applied to embed knowledge and
human experience in intelligent control algorithms. Decision making is
supported by a dynamic online help facility that can be easily maintained. The
comprehensive history database archives laboratory and process measurement data
which is transformed into a usable and easily understandable form with user
tools.
Project information
Participants
- Valmet Automation Inc.
- Metsä-Serla Oy, Kyro Board Mill
- Tekes
Project dates
Starting 1.11.1995 and ending 31.12.1996
Project volume
Total budget FIM 933.827
Project manager
Jukka Puhakka
Valmet Automation Inc., Neles Automation Group, Control
Systems
Lentokentänkatu 11
P.O. Box 237
FIN-33101 Tampere, Finland
Tel: +358 20 483 8035
E-mail: jukka.puhakka@nelesautomation.com
More information
Jukka Puhakka or
Timo Merikoski
Tel: +358 20 483 170
Fax: +358 20 483 84058405
E-mail: timo.merikoski@nelesautomation.com
11.2 Adaptive and intelligent systems in paper machine grade change
Abstract and goals
Grade
change on a paper machine or board machine means changing the current paper
grade to a new one. Grade change is made by changing machine variables such as
speed, stock flow and steam pressures at the same time to provide a fast
transition in quality variables such as basis weight and moisture. Because of
the complexity of the process the grade
change is very demanding operation.
The goal in
project was to investigate whether intelligent control and calculation methods
can be used to improve the control in the grade changes.
Methods and results
The fuzzy
control method was mainly studied in the project. Pilot research was carried
out on two Finnish production paper machines and results of the research have
been put into use.
Project information
Participants
- Prosessitutkimus H. Peltonen Oy
Project dates
1.4.1997 - 31.10.1998
Project volume
FIM 355.000
Project manager
Heikki Peltonen, DI
Prosessitutkimus Oy
Laserkatu 6
FIN-53850 LAPPEENRANTA
Tel. +358 5 6243 250
Fax +358 5 4120 949
11.3 Aggregate quality characteristics and
control of their effects with a neural network
Goals
- To recognize the most important concrete aggregate
quality characteristics and determine their impact on concrete in its fresh and
hardened state
- To be able to improve control the quality of concrete
aggregate already during its production and thus reduce the variation on
concrete as the end product
- To enable more efficient and economical use of the
aggregate pits/quarries in the company’s business area and in its strategic
expanding areas (Nordic countries and the Baltic Sea area)
- To test and learn the possibilities for using a neural
network to control the concrete raw materials and further extend its use in the
steering system for concrete production
Results and impact
- A Excel–based computer programme has been developed. By
entering values of the aggregate quality characteristics, it evaluates the
aggregate’s performance in the concrete. When also comparing the available
aggregates to the transportation distances one can choose the most economical
aggregate combination for concrete production.
- New, easy to perform and yet reliable testing methods
have been developed for aggregate production to gain information on the
aggregate quality characteristics.
- The neural network has been found to be a flexible and
useful tool to handle aggregate and concrete technology questions and problems.
- The aggregate and concrete producers feel more
confident when making decisions about aggregate selection. The use of
nontraditional aggregates, e.g. crushed and filler aggregates, have increased.
This extends the life span and economy of the aggregate pits/quarries, when
more of the raw material is used in the processed end use instead of as
unprocessed filling.
- The information gained in this project also helps to
direct aggregate production so that one can choose the best production
technique, e.g. normal production, washing, impact crusher etc. The best
economy is achieved when both the aggregate and concrete production costs are
optimized.
Project information
Participants
- Lohja Rudus Oy, aggregate and concrete producer, project
leader
- Partek Betonila Oy, precast element producer
- Lappeenranta University of Technology, neural network
programmer
Project dates
Starting date 01.01.1995, ending date 31.12.1997
Project volume
Total budget FIM 1.100.000
Project manager
Development manager Hanna Järvenpää
Lohja Rudus Oy
P.O. Box 49
FIN-00441 HELSINKI
Tel: +358-9-503 73 67
Fax: +358-9-503 73 96
E-mail: hanna.jarvenpaa@lohjarudus.fi
More information
Lohja Rudus Oy homepages:
www.lohjarudus.fi
11.4 Analyzer-based process control utilising neural networks and
fuzzy logic methods
Goals
The aim of the project was to develop a quality prediction model for the
cooking process in a pulp mill. The predictor was to be based on the
information received from a developed CLA2000 Cooking Liquor Analyser measuring
the alkali content, total dissolved solids and dissolved lignin during the
cooking process. Neural networks and fuzzy logic methods were to be the main
methods used in the development.
Results and impacts
Kappa number models (Kappa number = the main pulp quality variable in cooking)
for different types of digesters were developed. The models gave good results
when the feedback data from the final Kappa number was easily available. In
cases when the Kappa number samples had to be collected manually, the labour
required became too big, and other methods such as reference curves were found
to be more reasonable.
Other quality variables such as yield and pulp strength were also studied, and
similarly when the feedback data was available the results were promising.
However, the data needed to train the networks is not normally collected in the
mills, and generalisation of the achieved results remained as question marks in
this project.
The achieved results offer a better way to manage the cooking process control.
The developed quality predictor is a new product as such, and it also expands
the products range of cooking optimising software packages. In addition, it
improves the applicability of the CLA2000 Cooking Liquor Analyser.
The project also increased significantly the understanding of the methods used,
and at least some level of technology transfer from the university was
recognised.
Methods
Principal methods used were backpropagation and Levenberg-Marquardt neural network
algorithms and commercial fuzzy logic tools (Matlab). A short study was carried
out with linguistic equations. Conventional regression models and reference
curve methods were also used.
Project information
Participants
- ABB Industry Oy, Pulp & Paper
- University of Oulu, Control Engineering Laboratory
- Exens Development Oy
Project dates
Started: 1.1.1997, finished: 31.12.1998
Project volume
FIM 2.068.000
4Project manager
Raimo Sutinen
ABB Industry Oy
Systems Group
Tyrnäväntie 14, 90400 Oulu, Finland
Tel: + 358 10 22 52144
Fax: + 358 10 22 52140
E-mail: raimo.sutinen@fidri.mail.abb.com
Publications
Sutinen R., Prediction of cooking results using neural networks.
Automaatioväylä 5, 1996, (in Finnish).
Lemmetti A., Leiviskä K., Sutinen R., Kappa number prediction based on
cooking liquor measurements. Report A No 5, May 1998, Control Engineering
Laboratory, University of Oulu.
Murtovaara S., Lemmetti A., Sutinen R., Leiviskä K., Kappa
number prediction based on cooking liquor measurements. SPCI proceedings,
Stockholm. June 1999.
Lemmetti A., Murtovaara S., Leiviskä K., Sutinen R., Cooking
variables affecting the kraft pulp properties. Control Engineering Laboratory,
University of Oulu, 1999 (to be published).
11.5 Application of artificial neural networks in
the modelling of cold rolling and heat treatment processes
Goals
This project aimed to develop
neural network models by using measured process data from Rautaruukki's steel
strip production. The main goal was to combine all manufacturing processes into
one optimimizing model, using physical and neural models. The desired
properties, such as strength, formability and flatness, could be predicted and
controlled after each process stage.
Results
In the first stage, neural
networks were used to predict the properties of steel strip and to optimise the
process parameters in cold rolling. The second stage aimed to predict the
mechanical properties of the cold rolled products. Both of these stages have
been completed successfully.
Project information
Participants
The project was carried out in
cooperation between Rautaruukki Strip Products and the Laboratory of Materials
Processing and Heat Treatment at the Helsinki University of Technology.
Project dates and volume
his three year project was
completed on 30.6.1997 and the total budget was about FIM 2 million.
Project manager
Dr. Arto Ranta-Eskola
Rautaruukki Steel, Strip Products
FIN-13300 Hämeenlinna, Finland
Tel: +358 3 528 5467
Fax: +358 3 528 5620
11.6 Automatic tuning of intelligent controllers (ATIC)
Goals and results
The ATIC-project comprised of partner companies'
confidential R&D projects in which automatic tuning of intelligent
controllers was applied in different installations.
Under the umbrella of these separate projects all the partners
benefited from the experience gained in the different applications.
The versatility and increase in automation of machines and
processes have induced a strong growth in the number of the tuning parameters
of controllers. This type of development places increasing demands on the
knowledge and time of the user.
In order to reduce the period of starting up it is essential
to automate the tuning of controllers.
With automatic tuning the impact of users’ different views and
interdependencies on tuning parameters can be taken into consideration.
Usually the know-how about installations is very unexact and
empirical. This kind of know-how can be put to use with the help of fuzzy logic
while designing the controllers.
The aim in the R&D projects was not the generation of
new products but the improvement of the subsystems in large-scale
entities. The results have been
installed in commercial products such as drilling machines, harvesters, paper
machines, different kinds of service and diagnostic systems, to mention some
areas of concrete applications. It can
be said that ATIC projects have contributed to the competitiveness of partner
companies and led to several commercial applications.
Partners
- Neles Jamesbury Oy
- ABB Industry Oy
- Plustech Oy
- Tamrock Oy Technology Center
- Tamlink Ltd
- Valmet Automation Inc.
- Tampere University of Technology
- Helsinki University of Technology
Project dates
ATIC product development project was in progress for 3
years, from 01.03.1995 to 28.02.1998.
Project volume
The volume of the project was up to FIM 6 million.
Contact person / Project manager
Juha Leppänen
Tamlink Ltd
Hermiankatu 6
P.O. Box 140, FIN-33721 Tampere
Tel: +358-(0)3-3165 100
Fax: +358-(0)3-3165 123
E-mail: Juha.Leppanen@tamlink.fi
11.7 Comprehensive control of product and process information in
electronics production
Goals
The real-time information received from the devices on a
production line and the products being produced makes the observation and
control of the production line more comprehensive than at present. To arrive at
comprehensive observation and control, better management of the information
flood is needed. The information and knowledge, which are essential from the
point of view of the observation and control of electronics production, was
gained by combining product and process information. An evaluation prototype of
an online production monitoring system was tested in the project. The aim of
the evaluation prototype was to estimate the suitability of different methods
within the separate subsystems and identify a viable system architecture.
Results and impacts
In the project an evaluation prototype was developed. The
system applies fuzzy logic to processing data. The prototype produces a trend
of product quality indicators describing the situation of a product and line by
extracting and interpreting the data coming from single devices. At a glance the
trend gives operators an overall picture of the product and process quality
standard achieved on the monitored production line. The hierarchical
visualisation method, which has been developed for the system, makes it
possible to locate and predict the faults of the production devices and
products. The system remains open and easily modifiable. This is important,
because several products are often assembled on one production line, and the
measurements required by the control system may change not only between
products but also between different versions of a single product.
Methods
- Fuzzy logic
- Traditional statistical process control methods
Project information
Participants
- Nokia Mobile Phones
- VTT Electronics
Project dates
Starting January 1, 1999, ending November 31, 1999
Project volume
FIM 1.250.000
Project manager
Olli Nieminen
Salo, Finland
11.8 Development of a calibration method and new applications
[IMA QuarCon Analyser (XRF– bulk material analyser)]
Goals and results
IMA Engineering Ltd Oy
is a global supplier of advanced online xrf -analysers for different
types of industrial minerals processing plants.
IMA’s main customer group is cement plants and limestone
quarries. Typically, a cement plant can be considered as a vertically-integrated
industrial process from mine to ready cement, packed and delivered to final
customers. IMA products help cement customers to improve their operations; to
make savings in their raw materials handling, and to develop the process.
IMA has developed a distributed online bulk material xrf
-analyser system (called QuarCon) for analysis of limestone materials from the
conveyor belt for this specific customer group. In the first installations,
QuarCon analyser calibration proved to be complicated, and selected models were
unstable or had to be modified quite often. In addition, it became apparent
that at many quarries “the need to know” chemical composition as early as
possible is vital to improve the efficiency of the operation. In such cases,
QuarCon should be installed right after the first crushing stage, where lump
size is bigger, material bed fluctuations are higher, and generally the
environment is more harsh. It is
believed that such a situation is also typical in many base metal mines, so a
possible solution to this problem would mean new potential applications.
Parallel developments to improve the performance of QuarCon
were started in 1996:
- An automatic step-forward regression software prototype
was tested, and a study to find best commercial regression package was started.
- Empirical static and dynamic calibration methods were
studied in customer deliveries.
- Methods to measure and to compensate for material bed
fluctuations on the conveyor belt were studied.
- A new calculation method to eliminate or reduce the
error due to material fluctuations etc. was developed (today known as smart
IQCalc calculation software).
Step-by-step, new ideas and methods were tested in old and
new applications, and finally in late 1997, new methods, procedures and new
software proved out to give the desired result. The adopted method and
procedures include: special arrangement of irradiation and detection with
scattering channels to get more rugged and stable models, model selection using
a commercial regression tool (product name Statgraphics), procedures to perform
static calibration with model selection and testing, and procedure to perform
dynamic calibration and testing. Around the same time a new version of IQCalc
was developed, and this new smart calculation eliminates e.g. too low or high
tons or empty belt situation. Also, it is possible to use automatic model
selection, not to forget the possibility of combining and managing situations
with analysis results from several analysers and piles at the same time.
A new real-time platform (FIX from Intellution)
with Windows NT operating system with smart
IQCalc, IQReporter for easy reporting, and some tailored calculation and
control software also helped to reach the desired target with ease and speed of
calibration in new installations throughout 1998 and after.
After tests and head-to-head comparison with PGNAA method,
QuarCon was selected at the RTZ, QIT Fer et Titane project in Canada to analyse
titanium ore at their mine in Quebec. This new application and installation has
just been completed, and shall be reported in the international press soon.
This new application is expected to serve as a reference for base metal
applications.
Project information
Participants
The described development was possible only with close
cooperation with customers, who were interested in obtaining good performance
at new and older installations.
Project dates
The development project was officially started in early 1997
and ended in December 1997.
Project volume
The total cost was about FIM 1.5 million including estimated
work at customers plants.
Project manager
Mr Juha Kaikkonen, technical director
Tel. +358-9-8678 100
Fax: +358-9-86781020).
More information
IMA company and product information is available at the
Internet home page: www.ima.fi
and in the following publications: Halla Success with IMA,
International Cement Review 12/98, Calibration of on-line analysers,
International Cement Review 7/1998.
11.9 Dosing control of water treatment chemicals
Goals
The treatment of
industrial wastewater is a complex, non-linear process, which has long time
delays. In general, there are only few suitable on-line measurements.
So, the continuous dosing control of water treatment chemicals cannot be
optimised. The aim of this clinic project was to study the capabilities of
intelligent methods to control dosing.
Results
In this case, an industrial wastewater treatment process was
studied. The impurities of the water were measured as COD, suspended solids,
turbidity etc. The current dosing control of chemicals is simple. The dosage is
based on the flow rate of incoming wastewater. If the impurity level of
wastewater is constant, this control method guarantees the treatment result but
cannot prevent the overdosing at certain times.
owever, there are often great variations in the quality of water
to be treated. With a flow-related dosage of the chemicals the increase in
the impurities in the wastewater flow results
in insufficient purification. Another problem is that without any feedback
control the amount of chemicals cannot be optimised.
A lot of data was collected from the case process. The
neural network models were derived from this information. The target was to
teach the model predicting the turbidity of purified water on the basis of the
flow rate of wastewater, the amount of chemicals and the suspended solids in
the incoming wastewater. The MLP (Multilayer Perceptron)
models were developed with G2 NeurOn-Line studio software.
The results from the study are promising. The model learnt
the process rather well. However, the model should be improved and tested more
before it can be used for control purposes.
With reliable models, the process could be controlled so that the amount
of chemicals can be optimised.
There have been preliminary discussions about continuing the
work.
Project information
Participants
The work was carried out
in co-operation with Control Software Oy, University of Oulu and Kemira
Chemicals Oy. The software development was subcontracted by Control Software
Oy. The expertise of intelligent
methods was obtained from the University of Oulu.
Project dates
Project started in September, 1999 and lasted for about
three weeks.
Project volume
The costs were FIM 118.000.
Project manager
The project manager was Marjatta Piironen from Kemira
Chemicals Oy, Oulu Research Centre
(marjatta.piironen@kemira.com).
11.10 Finnotzo – air conditioning system for buses
Goals
The goal of the project was to develop temperature
regulation inside buses so that both air quality would be maintained and
temperature regulation would work with precision under changing conditions. One
of our targets was to remodel the unit in order to finish with the best
possible result in air circulation. Another important objective in our project
was to obtain the best possible raw materials and to use a production method
which enables us to speed up assembly.
The results and impacts
We have succeeded in developing a unit suitable for
serial production. We used subcontractors for some of the components, but the
rest were manufactured in our workshop, which results in shorter assembly
times. All this enabled us to give a positive response to short delivery time
requests. We have also been able to keep costs down. The unit has better
heating/cooling capacities than those of its predecessor, which has made finnotzo our customers’ choice for air
conditioning.
We have found a new method for manufacturing the base for the unit by adapting
vacuum forming of the plastic raw material.
We have also been quite successful in the development
of heating/cooling automatic controls. With the new automatic controls it is
far easier to control the internal temperature of a bus than with the old
system. With the help of these new automatic controls we have also been able to
improve the old controls.
During the project and thereafter we have acquired a lot of
new information in different areas of bus air conditioning. We have increased
cooperation with the bus bodybuilders in Finland. The cooperation has led to
constant improvments in the unit.
Methods
Modeling
The temperature regulation system in buses has been modelled
and the models have been experimented by carrying out test drives in varying
climatic conditions. A ”datalogger”-type measuring device has been used. Data
has been collected from various measuring points, for example the ambient
temperature, the internal temperature, the engine’s water temperature and the
opening of the magnetic valves on the heat exhangers. With the measured values
all the variables have been indentified and taken to the ASCET system, where it
has been possible to undertake simulations. All the information gathered this
way has been taken to the next level, where the information has been translated
into software.
Identification
We studied the temperature regulation process’s
basics and characteristics. We also studied and tested all convetional
regulation possibilities. Then we identified all the models and functions for
application of the fuzzy logic. The fuzzy logic was used to control heating
options and to control for exceptional circumstances.
Project information
Participants
Companies:
- Autojen Kylmälaite Oy
- Konsul Oy
- Xortec Oy
- Proautomatica Oy
- Muoviura Oy
Research institutes
Project dates
1.11.1996 – 31.12.1997
Project volume
Total budget FIM 900.000
Project manager
Timo Kauppinen
Autojen Kylmälaite Oy
More information
www.dlc.fi/autojen
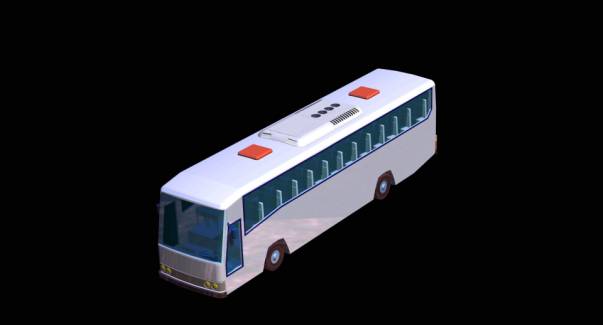
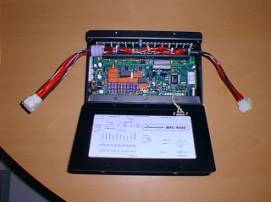
Figure 1. Finnotzo – air conditioning system for buses.
11.11 Fuzzy logic in Vacon frequency converters
Goals
The objective of the project was to use and develop fuzzy
strategies in the frequency converter. AC-drives are widely used in industry,
typically for cranes, elevators, paper machines, conveyors, winders, and pumps.
Fuzzy logic controllers were developed to control the
DC-link voltage of a voltage source inverter. The controllers are activated
when an over or under voltage situation appears. Over voltage in the DC-link
will appear when the frequency converter attempts to stop a high inertia load
(e.g. a large fan ) in a too short a time. The fuzzy logic controller seeks a
new deceleration time for the drive in such way that the DC-link voltage will
be kept constant. If the supply voltage is lost, the frequency converter has to
decelerate to maintain DC-link voltage high enough to keep the drive 'alive'.
In this case the frequency converter draws energy from the load.
Fuzzy logic was also used in the torque controller of the
Vacon frequency converter. Using fuzzy logic a more reliable product was
achieved which is important for industrial customers. Customer-specific
controllers and compensators were designed as well. E.g. the frequency
converter was used to give an actual value for an external analogue PID
controller inside the customer's hardware. Based on the current process state,
the actual value was modified by the fuzzy logic in the frequency converter.
The frequency converter was used to make a non-linear signal modification and
no PLC was needed. This feature caused the OEM customer to choose Vacon’s
frequency converter.
Project information
Participants
- Vaasa Control Oy
- Sigmateam Ky
Project dates
Project started on 01.02.1996 and ended on 31.12.1996.
Project volume
Total budget for the project was FIM 1,127 million.
Project manager
Veijo Karppinen
Tel: +358-6-212 1210
More information
Ilkka Laukkanen
Tel: +358-6-212 1296
11.12 Higher-level control system for lime kilns
Goals
The goal for the project was to develop a higher-level
control system using modern control methods such as fuzzy control and
multivariable control algorithms.
The main emphasis in process control was:
- to minimise emissions
- to optimise energy use
- to maximise the lifetime of refractories
- easier and more stable operation of the kiln
- to develop tools for remote operation follow-up.
Results
Fuel consumption of the kiln has been lower on average when
the control system
has been used compared to earlier statistics.
The reduction in emissions cannot be shown.
The lifetime of refractories is normally several years.
Temperature peaks that are harmful
for refractories have, however, disappeared.
The quality of the product, i.e. burned lime, is defined by
residual carbonate. Average residual carbonate has been higher as targeted and
the average deviation from the target has been smaller.
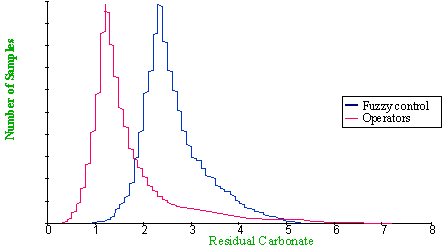
Figure 1. Change in residual carbonate deviation with help
of fuzzy control
Methods
The control system program was developed jointly with the
manufacturer of the DCS.
After testing it was loaded into the DCS of a Finnish pulp
mill. A test period of two months was arranged. The control system was not
running for the first 30-day-period. The second period was used to compare the
difference.
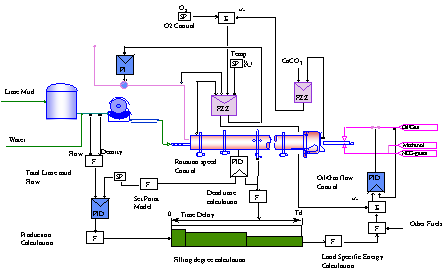
Figure 2. Lime kiln fuzzy control.
Project information
Participants
- Ahlstrom Machinery Corporation, Savonlinna, Finland
- Honeywell Oy, Center of Excellence, Varkaus, Finland
- Enocell Oy, Uimaharju, Finland
Project dates
Start 1.5.1996, end 31.5.1998
Project volume
Budget for the project was FIM 1.055.000.
Project manager
Keijo Savolainen, Director of Sales
Ahlstrom Machinery Corporation, Savonlinna
Phone: +358 15 5761.
11.13 Integrated neural network and hybrid systems modeller for the hot
rolling mill pass schedule calculation system
Introduction
A rolling mill pass schedule calculation system calculates the
settings for the rolling mill. The
system consists of process models and
several algorithms. The models are based on physical and experimental modelling
methods. Neural networks play a significant role in modelling because the
phenomenona in rolling are nonlinear and measured data is stochastic.
In this project the main goal was to develop a modelling
application that automates modelling, tests the models and gives statistical
information about the model
performance, such as std, rmse and
distribution. The application was implemented by using G2 software with
its NeurOn-Line and Diagnostic Assistant software tool kits.
Results
The application achieved by this project searches
automatically datasets from the rolling results database, scales and filters
data, trains and tests the neural network models and saves results and models
for pass schedule calculation system. It is also possible to simulate process
behaviour, optimise the structure of the model and generate and test hybrid
models (neural network models +
analytical models). A configuration tool (net builder) establishes
automatically the structure of the model (the number of nodes in hidden layer).
Simulations allow the user to generate some data to input into the model,
inspect the output generated from data and then save the results. The user can
specify the range (min to max) of values and number of increments for a given
input. The application displays the output data graphically.
The rolling force models for several types of steel were
implemented. The benefits of modelling with this application were more accurate
models and a more efficient modelling process than before. In the future it
will be possible to generate more general models by using hybrid models.
Methods
Multi-layer perceptron networks with back propagation
training algorithm were used for modelling. The net-builder
was based on the OLS-method. The models were
tested on independent datasets and testing results were analysed statistically.
Hybrid models were also tested during the project.
Project information
Participants
- Outokumpu Polarit Oy, Tutkimusosasto
- Control Software Oy
Project dates
Project starting date 1.1.1999, project closing date
15.3.1999
Project volume
FIM 100.000
Project manager
Harri Kiviahde
Outokumpu Polarit Oy
95400 Tornio, Finland
Tel: +358 16 453322
E-mail: harri.kiviahde@outokumpu.com
Pekkä Häyhä
Control Software Oy
Veneentekijäntie 4, 00210 Helsinki, Finland
Tel: +358 9 676744
E-mail: pekka.hayha@csoy.fi
11.14 Intelligent dosing control system
Starting status
PCE Engineering is a Finnish engineering corporation,
products of which include concrete technology systems and machines.
The production
is based on projects and products are customized to meet the needs of the
client.
Concrete consists of an aggregate material, water and
cement. The key factors in producing high quality concrete are mix design, the
finest raw-materials and their accurate dosing and proper temperature and
moisture of the concrete batch. This research project concentrated on finding
the correlation of these key factors and continued the previous research on
temperature and moisture control of concrete.
Goals and objectives
Earlier the accuracy of aggregate dosing has varied a lot
depending on the operator’s experience and knowledge of dosing parameters at
the batching and mixing plant. The goal of the project was to help the less
experienced operator to reach better concrete quality by more accurate dosing
of aggregates and better control of concrete temperature and moisture.
The first objective of the project was to develop a control
system which optimizes the dosing parameters and properties. The second
objective was to test and extend the temperature and moisture fuzzy control
systems to operate under more diffucult circumstances, i.e. at ready-mix
plants.
Results and impacts
The previous Elematic ProMix for Windows batching and mixing
plant control system has now been expanded to include also the new temperature,
moisture and dosing control systems. These control systems have been tested in
both winter and summer and they have performed successfully. According to test
results temperature, moisture and dosing controllability have been improved
despite the weather conditions and the operator´s experience.
Methods
The control systems were applied to the control of the
moisture, temperature and the accuracy of aggregate dosing. The control systems
were developed on the basis of
experimental measuring results and expert knowledge.
Project figures
Partners
- Tampere University of Technology
Schedule
The schedule was orginally from 1.1.1997 to 31.1.1998, but
it was extented to 31.12.1998.
Volume
The budget of the project was FIM 1.25 million.
Contact
Mia Kauhanen
Tel: +358 3 549 511
Fax: +358 3 549 5300
E-mail: mia.kauhanen@elematic.com
During Mia Kauhanen´s maternity leave (8.6.1998 – 2.8.1999)
Teemu Mattila
Tel: +358 3 549 511
Fax: +358 3 549 5300
E-mail: teemu.mattila@elematic.com
11.15 Method for measuring the liquid steel level
in a basic oxygen furnace (LD-converter)
Introduction
In a BOF, pig iron
containing about 4 % carbon is refined into raw steel by using oxygen lancing.
From a control point of view, it is very important to know the actual distance
between lance tip and hot metal surface. The traditional method involves
dipping a steel rod into the bath between two heats. This requires 5 to 8
minutes, which is a very long time relative to the actual blowing time (less
than 20 minutes).
Goals
The main requirements
set for the method to be developed were the following:
- Measurement range up to 15 metres
- Accuracy + 2 cm
- Ambient temperature 0 - 70 oC
- Reflecting metal surface, temperature 1300 to1600 oC
- If possible, also the slag/metal interface or thickness of slag layer
- Very dusty and vibrating environment
- Max. measuring time 2 minutes
- Connectable to process automation system
Results and Impacts
Tests at Fundia Wire Koverhar plant
A test series was
conducted in November-December 1997 at Fundia Wire Oy Koverhar plant. Koverhar
operates two 50-tonne BOFs. The radar was encapsulated in an enclosure made of
stainless steel.
The opening for the
lance and sublance was used to access the hot metal level.
The tests convinced us
that the microwave method gives consistently accurate information on the hot
metal surface, both before blowing and after blowing, plus the converter
bottom.
A permanent installation
on both converters at Koverhar was carried out in 1998.
Main impacts
Technically it is now
possible to measure the bath level for every heat. Exact bath level information
is passed to the process automation system which, among other things, controls
the lance and oxygen blow rate. The benefits of the new method are:
- Savings in converter lining costs
- Improved steel yield because of decreased iron losses to the slag and
because of decreased slopping
- Improved productivity due to the shorter measurement time
- Less variation in end-point carbon and temperature
- Improvement in working conditions
Methods
Microwave radar
It became obvious that
the microwave radar, tested already in the 70s, is perhaps the most suitable
method [1]. The test radar by Krohne was a continuous wave device working at a
10 GHz frequency band. Setting of parameters and processing of measurement
information is carried out by software. The basic software classifies the
echoes and selects the strongest signal representing the air/metal interface.
The device is equipped with a PC CAT interface for connection to a PC. For
connection with the process automation system a standard 4 to 20 mA interface
is used.
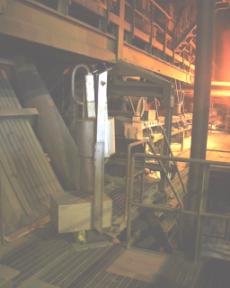
Figure 1. Microwave
radar based measurement system at Fundia Wire Koverhar Plant.
Project information
Participants
- Finx Oy (coordinator)
- Fundia Wire Oy Ab (steel manufacturer)
- Euroquest Oy (algorithms)
- Imamic Oy (radar technology)
Project dates
Start: August 1, 1997, finish: February 28, 1998
Project volume
Budget: FIM 118.000
Actual: FIM 107.700
Project manager
Erkki Saarelainen
Finx Oy
Revontulentie 6
FIN-02100 ESPOO, FINLAND
Tel: +358-9-4351602
Fax: +358-9-4351603
E-mail: erkki.saarelainen@finx.fi
More information
Please contact project manager or visit our web page
http://www.finx.fi .
References
[1] Kari Leppälä: Development of hot metal level measurement
for LD converters. Thesis for Engineering Degree, Helsinki University of
Technology, 1975 (in Finnish).
11.16 Model-CC: neural network technology for
process modeling
Goals
To develop a product which uses neural network technology in
process modeling and optimization.
As a result of the project a new process modeling tool, the
Model-CC quality control system, has
been developed. This system has been used in pilot tests in several pulp and
paper mills. The Model-CC system has
used to predict the quality variables of the end product. In some cases it is
also possible to obtain a recommendation for control action. The Model-CC
system is not used in a closed control loop. The main goal when implementing
the system in the mill was to reduce the amount of broke.
At the moment there are two online projects in the
implementation phase. In one project, the Model-CC system is being used to
reduce the grade change time in a paper machine. The Model-CC system’s user
interface is implemented on the display of the process automation system.
Before the grade change the system makes a recommendation for the nip pressure
of nip rolls and thus reduces the time delay when
paper thickness is in quality tolerance.
In the other project, three quality variables are being
predicted. The Model-CC system is used to give a control recommendation if any
of them goes out of quality tolerance.
Methods
The Model-CC system is built up from several commercial
products, including a database, a neural network program and data acquisition
programs. The specifications, data acquisition drivers, tools for maintaining the
system and user interfaces are produced by Control Software Ltd. Predictions of
quality variables and recommendations for control actions are based on neural
networks models. In most implementing projects emphasis is on collecting
process data and process knowledge in cooperation with the customer’s
personnel.
Project information
Project was started by Corintec Oy. The business was sold to
Control Software Oy on 1.11.1998.
Participants
- Euroquest Oy, Lappeenranta
- Taipale Engineering, Lappeenranta
- Enso Publication Papers Oy, Anjalankoski
- Laminating Papers Oy Ltd, Kotka
- Metsä-Botnia Oy, Kemi
- Metsä-Rauma Oy, Rauma
- Valmet Paperikoneet, Pansio
Project dates
Starting 2.1.1996, ending -
Project volume
FIM 4.184.393
Contact information
Pekka Ruusunen
Control Software Oy
Veneentekijäntie 4
FIN-00210 Helsinki, Finland
Tel: +358 9 676744
Fax: +358 9 670077
E-mail: Pekka.Ruusunen@csoy.fi
11.17 Neural networks for material properties
Goals
Thermodynamic
properties of substances are needed in numerical simulation of industrial
processes, e.g. in process and control design, safety analysis, and operator
training. Properties that are often required include temperature, density, mass
fractions, enthalpies, and partial pressures under different process
conditions. Rapid calculation of these properties is also necessary in the
measurement of several process variables needed for process control in
distributed control systems (DCS).
Conventional
algorithms for the calculation of thermodynamic properties typically consist of
several iterative steps including calls to complex functions and interpolations
in tables based on both theory and experimental data. The iterative algorithms
are too slow to be used in dynamic process simulators that should run faster
than real time. Methods based on tables and linear interpolations are fast, but
in practice limited to cases with only two or three inputs.
The goal of
this work was to study the feasibility of using neural networks (NN) to
calculate the material properties of substances.
Results and impacts
A total of
7 neural network models were identified for different phases of H2O
(Fig. 1). The preset accuracy limit of 1% of the output range was achieved in
every point of the training and validation sets.
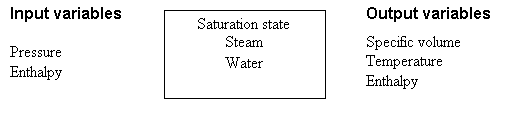
Figure 1. Schematic representation of neural networks for different
phases of H2O.
The NN
models proved to be considerably faster than the conventional series
development approximations that usually are applied to H2O.
In typical test cases, the NN models were
only 15 times slower than linear interpolation used in the dynamic process
simulation program APROS. However, the number parameters of the identified
networks were 800 times smaller than the breakpoint values for linear
interpolation. Linear interpolation is feasible only when the number of inputs
is one or two. The NN models can also be used in higher dimensional cases.
These
results showed that NN models are useful for the description of thermodynamic
and other material properties of substances when the number of inputs is higher
than two. Only few parameters need to be stored and the calculation is rapid
enough for dynamic simulation.
Based on
this study a new project “Modeling of thermodynamic properties of substances by
neural networks” started in March 1998. In that project a general method was
developed for the generation of fast neural network models approximating the
thermodynamic properties given by existing methods.
Methods
Feedforward
networks with one hidden layer of sigmoid neurons were used throughout the
work. The chosen multilayer perceptron (MLP) network structure has been
theoretically proven to be capable of approximating any function having a
finite number of discontinuities with arbitrary accuracy. The generalization
feature made it possible to train the network with a representative set of
input-output pairs and then also achieve sufficient accuracy with new inputs of
the validation set. Use of the same neural network architecture in all cases
made it possible to develop a general training procedure. The
Lewenberg-Marquardt optimization algorithm was used for the estimation of the
weights and cross-validation for choosing the number of hidden neurons.
Project information
Participants
- Finntech Finnish Technology Ltd
- VTT Automation
Project dates
1.4.97 – 30.05.97
Project volume
FIM 100.000
Project manager
Osmo Nojonen
Finntech Finnish Technology Ltd
P.O. Box 402, FIN-02150 Espoo, Finland
Tel: +358 9 456 6148
E-mail: osmo.nojonen@finntech.fi
Publications
R. Lilja and J. J. Hämäläinen (1999), Modeling of
Thermodynamic Properties of Substances by Neural Networks,
Proc. International Joint Conference on neural Networks (IJCNN '99),
Washington, DC, July 10-16, 1999.
11.18 Neural networks in the control of blast furnace processes
Goals
The aim of the project was to study the applicability of
neural networks for the control of blast furnace processes. The main goal was
to develop neural network applications which can be used in controlling the
horizontal and vertical temperature profiles as well as the horizontal gas
distribution profiles of the blast furnace.
Results
At the beginning of the project the existing neural network
applications in iron and steel industry were examined. The current status of
knowledge was mapped by these investigations. This knowledge formed a basis for
further development in this project.
New neural network based applications were developed for the
monitoring and interpretation of blast furnace wall temperature and gas
distribution measurements. These applications were implemented in the
Rautaruukki Raahe Steel’s blast furnaces. Operating experiences have been
positive and applications are utilised daily in the process control. In
addition, a system for the monitoring and interpretation of signals from the
blast furnace stockrods was developed.
Based on the result of this project, Rautaruukki Engineering
has develop a Blast Furnace Neural System, or BFNS for short, which makes it
easier to observe and handle profile data on vertical blast furnace lining
temperatures and horizontal temperature/gas analysis profiles. The BFNS
consists of on line neural network applications, which classifies the measured
blast furnace data into one of the predetermined classes. The results are shown
online on special displays (Figure 1). From these displays operators can
observe the types of profiles and how they have changed during the selected
viewing period. The BFNS also contains an environment for training and testing,
which can be used to update online applications whenever needed.
The BFNS forms a new desired link between charging and blast
furnace performance. The main benefit offered by the BFNS is an improved utilisation
of the blast furnace wall temperature and gas distributions, which means more
accurate and reliable process control. The BFNS has been taken as a new product
for Rautaruukki Engineering, which is a business unit of the Rautaruukki Group.
The first implementations of the BFNS outside Rautaruukki Steel were made in
1999.
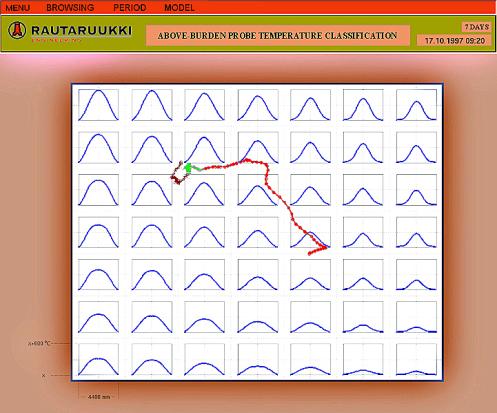
Figure 1. An Example of a Blast Furnace Neural System
display.
New projects relating to neural networks, genetic algorithms
and fuzzy logic have been started as a result of this project.
Methods
Both supervised and unsupervised neural network
architectures were studied in this project. It was observed that neural network
models using supervised learning are effective when features of process data
can be easily extracted. Neural networks using supervised learning are useful
whenever it is possible to define input/output patterns but when it is not
known exactly what is between them. The power of unsupervised learning methods
is their ability to extract the basic features of data. Therefore they are
especially suited to situations where extraction of typical features from a
large and complicated dataset is required.
Performance of the neural network models was evaluated
against various statistical models. These evaluations proved that there are
many difficult tasks of this type where it is very difficult or even impossible
to develop a good model with conventional methods but where neural network
models are very powerful.
Project information
Participants
The project was carried out in cooperation between Finnish
Universities and Rautaruukki Co.
Project dates
Project started in autumn 1995 and it concluded at the end
of 1998.
Project volume
The total budget was about EUR 1.2 million.
Project manager
Martti Miettinen
Rautaruukki Oyj Engineering
www.rautaruukki.fi/Engineering.
11.19 Opportunities offered by mathematical modelling at Rautaruukki
Steel Strip Products
Goals
The objective of this project was to evaluate mathematical
modelling possibilities in strip production, and identify those that are likely
to result in clear benefits with small effort, cost, time and risk. This survey
was also to result in a broader picture of mathematical modelling possibilities
that could be considered and where the new ideas fit in. Ideas from this
project are likely to result in significant benefits.
Mathematical modelling is an important part of the research
and development activities at Rautaruukki. Rautaruukki focuses on higher level
automation as one of the important activities in corporate research.
Mathematics is often at the core of automation systems. Therefore it is
important to have a good idea of what can be achieved and what needs to be
done. This project was expected to result in a better view of the possibilities
of mathematical modelling in strip production. This expectation has been
fulfilled.
There is virtually no limit to the possibilities of
mathematical modelling in strip production. This work has therefore focused on
realistic possibilities, particularly those which are either seen as needs or
as good ideas worth considering. The ideas have been gathered during
discussions with personnel at Rautaruukki Strip Products division, as well as from
literature.
Results
The results of the project are the ideas and an evaluation
of their value. Most of the information has been recorded in the form of lists,
which are divided into five areas:
- Short descriptions of the ideas that were mentioned during the
project.
- A list of ideas without descriptions.
- A list of the participants, and the two evaluation committees
which commented on the ideas.
- The packages of the ideas. Many ideas were related and
overlapping, so at a certain stage some ideas were fused together into
packages.
- A list of the better ideas. The list reflects the opinions of
the author of the report, and takes into account primarily the comments of the
two committees, but also an overview of the ideas in the list, their potential
to Rautaruukki, the technical risks, the size of the possible projects and
available technical background and/or production data. The list can be expected
to have a slight bias in favour of empirical modelling.
>
Project information
Participants
- Rautaruukki Strip Products
- Abhay Bulsari, AB NONLINEAR SOLUTIONS OY
Project dates
November 1998 - April 1999
Project volume
The total budget was FIM 50.000.
Project manager
Dr. Arto Ranta-Eskola
Rautaruukki Steel, Strip Products
FIN-13300 Hämeenlinna, Finland
Tel: +358 3 528 5467
Fax: +358 3 528 5620
11.20 Paper web break sensitivity indicator (PAJE)
Goals
This
project has developed a paper web break sensitivity indicator for a paper
machine. This indicator(s) is based on analysis of the process measurements online
in order to make current predictions of paper web breakage propability. This
information can be used to direct actions of the operators and maintenance
personnel to identified problem areas.
Paper web
breaks are one of the three factors affecting on the net efficiency of a paper
production line. Paper web breaks cause about 3% efficiency decrease in a paper
machine production. The other two are shutdowns and produced broke amount
corresponding about 4% and 6% of the efficiency decrease, respectively. These
figures are highly process and machine dependent.
It has been
estimated that 1% increase in net efficiency increases the annual profit of a
large paper machine by $ 1.5 million.
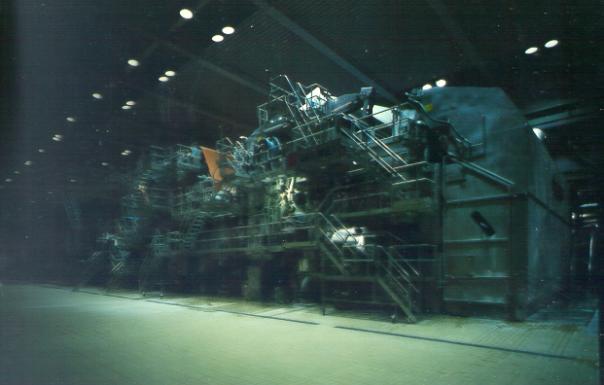
Figure 1. The wet end of a paper machine.
The project
has produced 2(+1) independent paper web break sensitivity indicator versions.
The
development of these indicators has required methods to manage the vast amount
of process measurement data covering long time periods. The requirement to be
able to combine process measurement data with other information sources and to
analyze them together has lead to practical solutions, e.g. a visual reporting
tool. A data collection and processing process is also defined and has been in
use in the project.
This
project has been working in paper making process area that cannot currently be
controlled well enough. The project has developed tools to handle process
measurement analysis online. Research institutes have learned more about the
process and they have developed their tools and knowledge accordingly. The
paper manufacturer UPM-Kymmene has been able to utilize the results of this
project’s web break analysis and reporting tool. With this tool they can
accurately identify where in the machine the breaks are occurring over a long
time and are thus able to direct their maintenance activities. Although the
problem of web breaks is not completely solved, the need remains for tools
which help to run a paper machine more economically.
Methods
The project
has developed 2 (+1) independent indicator versions. These different versions
operate using different principles: one version operates as a Case Based
Reasoning (CBR) system. This indicator version compares the current process
measurement state to several state samples representing different amounts of
web breaks. The closeness of the current state to samples relates to the web
break sensitivity of each sample. This approach is using the linguistic
equations method developed by Esko Juuso.
The second
approach is based on univariate signal feature extraction and following
preprocessing perceptron network. Each process measurement is analyzed to
identify features whose existence and magnitude tells how much that signal is
behaving in a risk-increasing way. The training phase adapts a sigmoidal
function to each process measurement to make a separation between
risk-increasing and risk-reducing features. In the analysis phase, separate
signal risk factors are summed up to give a single risk figure. The process
delays and signal setting times are taken into account yelding a risk figure
that is related to time.
Project information
Participants
- Valmet Corp.
- UPM Kymmene Corp.
- Oulu University, Department of Process Engineering
- Åbo Akademi, Institutionen för Informationsbehandling,
- Helsinki University of Technology, Control Engineering Laboratory
- Tampere University of Technology, Automation and Control Institute
- General Electric, Corporate Research and Development
Project dates
1.9.1995 – 30.12.1999
Project volume
FIM 5 million
Project manager
Kari Oinonen
Valmet
P.O. Box 587
FIN-40101 Jyväskylä, Finland
Phone: +358 20 482 6194
Mobile: +358 40 733 8908
E-mail: Kari.Oinonen@valmet.com
Publications
Timo Ahola, Jari Ruuska, Esko Juuso and Kauko
Leiviskä/ Web Break Sensitivity Indicator, in Proceedings of TOOLMET ’99
Symposium- Tool Environments and Development Methods for Intelligent Systems,
Oulu, 1999, pp. 202-209.
Patrik Eklund, Tony Riissanen/ Forest Informatics, IFORS
SPC-2 Conference, 25-27 April, 1999, Turku, Finland, pp 26-29.
P. Eklund, J. Zhou, Comparision of learning strategies for
parameter identification in rule based systems, J. Fuzzy Sets and Systems, to
appear.
11.21 Prediction of electricity consumption
Goals
The opening of National Electricity Stock Market (EL-EX) a
few years ago made possible for electricity companies to trade their own
production easily with other companies and large-scale consumers. Prediction of
future consumption in few next day scale can give a great advantage in both
purchasing and selling electricity.
The aim of this project was to study the possibility of
predicting consumption in particular distribution area using neural networks. A
further aim was to design PC-software based on neural networks to help in the
calculation and simulation of different conditions.
The project is still continuing with private financing. The
overall aim is to investigate the markets for software and to develop software that
provides a convenient tool for estimation of consumption.
Analysis of different neural networks eabled the
identification of the optimal network design. However, the difference between
the results and realization must be quite small (less than 5 percentage units).
The best model so far gave difference of between 0 to 8 % and an average
difference of 4.5 %. Considering that consumption in Finland varies very much,
result was satisfactory.
Methods
Further information about the software design methods is
provided by Tommi Laitinen and neural network design by Ossi Taipale.
Project information
Participant organisations
- HALT Ohjelmointi Oy, software design, project coordination
- Taipale Engineering Oy, neural network design
- Muotoilutoimisto P Haiko, user interface
Project schedule
1.2.1997-31.8.1998
Project volume
FIM 220.000
Project manager
Tommi Laitinen
HALT Ohjelmointi Oy
Perillistenkatu 1
53100 LAPPEENRANTA, Finland
Gsm: +358 40 589 7023
E-mail: tommi.laitinen@halt.net
11.22 Prediction of nozzle clogging - a neural computing approach
Introduction
Clogging of nozzles is a problem metallurgists have been
familiar with for a long time. Until today, no definitive solution to the
problem has been found.
Typically, nozzle clogging occurs during casting of low
silicon aluminium-killed steel grades. A submerged entry nozzle (SEN) is used
in transferring liquid steel from the tundish to the mold, in order to
eliminate contact between the steel and atmospheric air. The material of SEN is
either SiO2 or alumina-graphite, depending on the manganese level of
the steel grades. Alumina compounds are usually accumulated at the upper end of
the SEN, close to the stopper rod tip, or alternatively at the lower end of the
SEN [1].
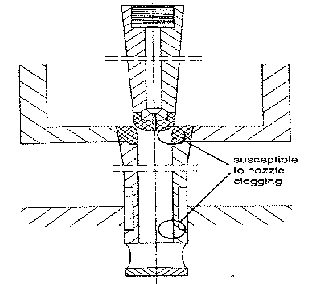
Figure 1. Areas of nozzle clogging in the SEN [1].
Clogging also occurs when using calcium treatment to improve
castability via oxide modification. Precipitation of CaS is a function of the
calcium, oxygen and sulphur contents of the steel [1]. Metallurgically,
clogging can be avoided, if the inclusions in the steel can be kept liquid
during casting, i.e. within a certain chemical composition range. In practice,
this has been the method used to prevent clogging.
Imatra Steel
Imatra Steel is a producer of low alloy special steels for
European automotive and engineering industries. The product range covers low
alloy engineering steel bars, squares, flats and forged vehicle components for
motors, transmission and suspension, as well as leaf springs and tubular
stabilizer bars.
The melting shop of Imatra Steel Works consists of one 75
tonne electric electric arc furnace, a secondary metallurgy plant, a two-strand
bloom caster as well as a reheating furnace for hot bloom charging. The blooms
are rolled at a breakdown mill into heavy round bars or billets to be rerolled
into round bars and flats.
Imatra Steel’s bloom caster is equipped with a mold level
control system which is based on radioactive mold level measurement with Co60and
fuzzy logic technology. The system detects on-going clogging of nozzles and
starts automatic counter-measures.
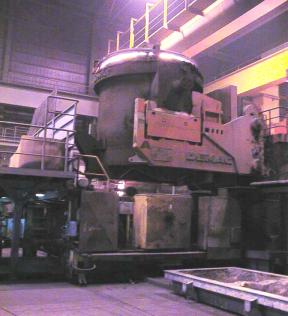
Figure 2. Two-strand bloom caster at Imatra Steel.
Study objectives
- To test whether neural computing would be applicable to
the nozzle clogging problem.
- To achieve a better knowledge of process variables
affecting to the tendency to clog.
- To find effective measures to be taken at a secondary
metallurgy facility in order to avoid clogging in individual heats.
Implementation
Retrieval of data
The total amount of different steel grades produced at
Imatra Steel is about 300, grouped into ten main families. For this study a
production data set for 2000 heats was gathered. The data is resident on the
plant’s main database computer. The data was from the year 1997, after
commissioning of a new arc furnace. The steel family M-steels (M = steels with
improved machinability) is relatively uniform and was therefore selected as a
target group for the study.
Selection of process variables for the model
The best neural network model was achieved by selecting the
variables based on the steel making expert at the mill. The prediction accuracy
advanced significantly compared to the results of mathematical data analysis.
The number of the process variables was reduced from 58 down to 8 (Table 1).
Table 1. A list of input variables.
Calcium content
|
Sulphur content
|
Soluble aluminium content
|
Temperature of incoming ladle
|
Tundish temperature at 1/3 point
|
Casting speed at 1/3 point
|
Total aluminium
|
Liquidus temperature
|
Two output variables
in the neural model were defined; namely clogging in the ladle slide gate and
clogging in the SEN.
Data analysis
A careful analysis for the data to be used in the models was
carried out. The analysis included mostly manual checking of validity,
correction of human errors, removing records which included missing values etc.
Thus it was made sure that the conformity in data was at a good level. In
several neural computing projects it has been realized that a high quality of
data is one of the key issues.
Cluster analysis
The quality of the data for classification was studied using
cluster analysis in order to determine whether clear groups could be found.
Data distributions between input and output variables were analyzed, both for
actual clogging and non-clogging samples.
The analysis confirmed that function fitting probably gives
a better model than classification. The clogging phenomen is
highly non-linear.
Final model
The final model was based on 311 M-steel heats. Of those, 29
heats were observed to have actual clogging in the SEN area. The training data
set consisted of 350 first heats.
Discussion of the results
General models vs single grade models
The correlations were clearly better for a single steel
grade data than for a family of grades. This directed the neural network
training work for single grade modelling. It was experienced that the best
models can be achieved using single grade data. The behaviour from one grade to
another was too different to be described in the general grade models. In other
words, it was not possible to obtain sufficiently good relationships between
the process parameters and the nozzle cloggings.
In data analysis of multigrade the correlations between the
input variables, measurements were small. The dependencies between the
variables were attenuated each other because of the difference between the
behaviour of different grades. When the data analysis and the models were
performed for one grade at a time, clear and good results were seen.
Testing of trained neural network
The reliability of the trained neural network was tested by
allowing the neural network to predict cloggings, using test data never “seen”
by the network. Reliability is measured by the difference between actual
cloggings and predicted values.
Based on our experience in this project the "unseen
data test" remains best way to see the reliability of the neural network
model.
Achievement of goals
- Neural network technology can be successfully applied to
the nozzle clogging problem.
- The prediction capability is at acceptable level for
practical purposes, 27 out of 29 cases.
- The countermeasures against
clogging can be taken at a secondary metallurgy plant.
Future development
A future
study is planned to establish the performance of the neural network in
comparison to a conventional method. The latter would be based on the actual
steel analysis and evaluation of the dominant state of the
inclusions in the steel, either solid or
liquid, at the casting temperature.
To develop the network further to a production version, an
analysis of the role and importance of two of the parameters, tundish
temperature and casting speed, is also needed.
11.23 Raw material management from quarry to kiln
[IMA Quality Control System (QCS)]
Goals and results
IMA Engineering Ltd Oy
is a global supplier of advanced online xrf -analysers for different
types of industrial minerals processing plants.
IMA’s main customer group consists of cement plants and
limestone quarries. Typically a cement plant can be considered as a vertically
integrated industrial process from mine to ready cement, packed and delivered
to final customers. IMA products help cement customers to improve their
operations; to make savings in their raw materials handling, and to develop the
process. During past 4 years, IMA has focused in developing a distributed
online xrf-analyser and a raw material management system for this specific
customer group. This vision or concept
includes two different types of online xrf-analyser modules and analysis
calculation and specific control software modules, to manage raw material flow
from quarry to kiln. This total technical system or concept is called the IMA
Quality Control System (QCS).
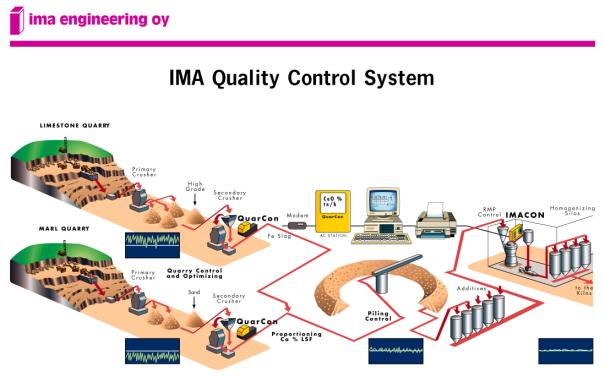
Figure 1. The IMA distributed raw material management system
may have several analysers connected with the same system.
There are two different online xrf-analyser product
families: QuarCon Systems 200 and 400 for bulk material analysis from a
conveyor belt, and IMACON 10 and 100 Systems for fine raw materials. The
software modules include a commercial real-time platform (FIX from Intellution), the Windows NT operating
system and some optional modules. IMA has developed the following software
modules: IMACON and QuarCon drivers to integrate 8 analysers in the same
system, smart IQCalc analysis and average calculation software (with a
Paradox-type open database), IQReporter for easy reporting of instant, average
or cumulative results, intelligent RMPCalc Raw Mix Proportioning software to
control especially the kiln feed materials, and some tailored calculation and
control software.
IMA QCS system architecture and software modules have been
developed step-by-step along with the
developments in the two abovementioned analyser families. New features and
software modules have been included in new deliveries and occasionally in
system upgrades. Development continues and new products or features will be
available in coming months or years.
Project information
Participants
Such large scale development has been, and will be, possible
only with close cooperation between sub-suppliers, customers or strategic
partners. As an example, General Electric R&D has played a key role in
RMPCalc fuzzy logic controller design, which eventually led to marketing and
sales cooperation with GE Industrial Systems in the USA and Germany.
Project dates
The development of the IMA QCS project started in August
1996 and ended in December 1999 with the current specification.
Project volume
The total cost is several million FIM.
Project manager
Mr Jukka Raatikainen, managing director
Tel. +358 9 8678 100
Fax +358 9 86781020
More information
IMA company and product information is available through their
Internet home page: www.ima.fi and in the
following publications: Raw Material Management, International Cement Review
5/1998, or Halla Success with IMA, International Cement Review 12/98. Coming
soon: Raw Meal Control, World Cement 6/99 and IMACON new tool for Raw Meal
Control in International Cement Review 6/99 .
11.24 Software toolkit for design of fuzzy
applications in industrial automation
Goals
Fuzzy
computing and fuzzy control have potential in various automation tasks where
sufficient performance is not achieved with conventional methods. Applications
can be, for example, control loops and computing tasks where nonlinear
characteristics must be taken into account.
The goal of
the project was to develop a software tool for the company. With the tool,
feasibility studies of fuzzy computing and the design of fuzzy systems can be
carried out in a flexible way. When the software tool has been created, a
service package can be developed and marketed for customers.
Results and impacts
The design
software makes it possible to create fuzzy systems based on both experts' knowledge
and process data by utilizing optimization methods. Different models can be
compared and analyzed flexibly with the software.
As of the end
of May 1999, the development of the software tool has not been finished.
However, the toolkit had already been tested in practice and the results had
been promising. Pilot research projects and tests were carried out during the
project at two Finnish paper mills.
In future
projects, the developed software toolkit will be one of the company's basic
tools. Due to increased efficiency that the toolkit provides it is assumed that
the productivity of the company will be increasingly improved.
Methods
The
software toolkit is developed in the MATLAB environment. Computing functions
are integrated into a graphical user interface.
Project information
Participants
Prosessitutkimus H. Peltonen Oy
Project dates
1.8.1998 - 31.12.1999
Project volume
FIM 353.200
Project manager
Heikki Peltonen, DI
Prosessitutkimus Oy
Laserkatu 6
FIN-53850 LAPPEENRANTA; Finland
Tel. +358 5 6243 250
Fax +358 5 4120 949
11.25 Temperature and moisture control of concrete with fuzzy control
Starting status
PCE Engineering is a Finnish engineering corporation, the
products of which include concrete technology systems and machines. The
production is based on projects and products are customized to meet the needs
of the client.
Concrete consists of aggregate material, water and cement.
The key factors in producing high quality concrete are the mix design, the
finest raw-materials and their accurate dosing and the correct temperature and
moisture of the concrete batch. This research project concentrated on finding
the correlation between these key factors.
Goals and objectives
The goal of the project was to control concrete temperature
and moisture during the manufacturing prosess. The aim was to develop a fuzzy
controlling system which keeps the temperature and moisture of a concrete batch
stable and constant as required by the operator. The new system calculates the
heating times for aggregate silos automatically and gives temperature and
moisture estimates for the aggregate materials in the silos. The control system
of the baching and mixing plant then calculates the necessary amount of cold
and hot water to reach the target temperature and moisture content of concrete.
Results and impacts
The temperature and moisture fuzzy controlling systems
developed in this project were tested at an element factory. According to the
test results, both temperature and moisture controllability have been improved
regardless of the weather conditions and the operator´s experience.
Methods
The fuzzy control systems were applied to the control of the
moisture and temperature of concrete. The control systems were developed on the
basis of experimental measurements and expert knowledge.
Project figures
Partners
- Tampere University of Technology
Schedule
The project schedule was orginally from 1.2.1995 to
31.12.1995, but it was extented to 31.12.1996.
Volume
The budget of the project was FIM 1.8 million.
Contact
Mia Kauhanen
Tel. +358 (0)3 549 511
Fax +358 (0)3 549 5300
Email: mia.kauhanen@elematic.com
During Mia Kauhanen´s maternity leave (8.6.1998 – 2.8.1999)
Teemu Mattila
Tel. +358 3 549 511
Fax. +358 3 549 5300
Email: teemu.mattila@elematic.com
11.26 Tool for realising fuzzy control in
commercial SCADA systems
Goals
The main goal of this feasibility study was to specify a set
of software applications for a flexible fuzzy system environment that could be
linked to an existing automation system.
The target was to specify structure and features of this new
fuzzy logic software tool having especially following properties:
- Hardware and software independent as far as possible
- Ease of use
- Flexible implementation
- Versatile and intelligent (adaptive) controller algorithms
- Easy to tune for various processes
With the help of experienced personnel at the Control
Engineering Laboratory, the main features and application tools were specified
to achieve the requirements. In addition, some interesting ideas were suggested
to strengthen the advantages and minimise the complexity of fuzzy systems.
As a result, a number of variations and requirement
specifications were outlined for a new fuzzy logic software tool. The decision
about continuing the project will be made later by the company directors.
The first interesting and challenging implementation for the
fuzzy control tool was planned to be, for instance, a multivariable and
unlinear biotechnical process.
Methods
During the study, it became clear that no individual
software development tool could solve the tasks alone. Therefore, the following
tools and methods were used in research and test work: Fuzzycon, FuzzEqu &
NeurEqu, MatLab, FuzzyTECH and DataEngine.
Project information
Participants
- Kaarlenkaski Oy
- University of Oulu, Control Engineering Laboratory
Project dates
1.3.1997 - 9.10.1998
Project volume
FIM 100.000
Project manager
Tapio Myllykoski
E-mail: tapio.myllykoski@kaarlenkaski.fi
More information
Kaarlenkaski Internet homepage:
www.kaarlenkaski.fi
11.27 Use of a neural network to control a separation process
Goals
The goal of this project was to test whether a neural
network program is suitable for the simulation and control of the
chromatographic separation process. A simulated moving bed process was selected
for the application to be tested. Only certain key parameters were selected to
be controlled by the neural network model.
Results and impacts
A neural network model was developed to simulate the
chromatographic moving bed process of glucose and fructose. The developed
neural network model is able to simulate the process by calculating the dry
substance profiles based on feed load and on some cycle step volumes. The
developed model can be also used for the optimization of parameters based on
the target performance.
The model has been tentatively used for offline process
optimization. The model is estimating well the parameter changes needed. By
using the model it would be possible to achieve an even and improved process
performance. No online control or optimization tests have been done. After this
Tekes project additional calibration data has been collected. It is obvious,
that a neural network model could be used for the mill scale process simulation
and optimization also for other chromatographic separation applications.
Methods
To calibrate of the model, mill scale data were collected by
using laboratory analyses of the samples and statistical methods for test
plans. A neural network model was developed by PC-based Matlab programs to be
used by PC. The neural network model was made in cooperation with Taipale
Engineering Ltd.
Project information
Participants
- Cultor Oyj
- Taipale Engineering Ltd
Project dates
October 1994 – October 1996
Project volume
FIM 441.000
Project manager
Heikki Heikkilä
Cultor Oyj, 02460 Kantvik, Finland
11.28 Use of a neural network in the quality control of particle board
production
Goals
The aim of Puhos Board Oy
was to clarify, whether it is possible to improve the quality control of
production by using a simulating model based on a neural network.
Detailed goals were:
- To improve the data collection from the production
- To find the most important data and also the meaningless data
- To find out most sensitive parts of the process
- To find out the best solution in using the existing
wood material for different board types
- To minimise the cost of changes from one board type to another
- To have information for changing the process during
investments
Results and impacts
During the project the process was analysed carefully and
the process knowledge of the personnel increased. Trend analysis has shown a
good correlation between the model and measured values. Most of known rules of
particle board technology are similar to those suggested in model. New rules
have also appeared.
The potential of modelling has been demonstrated and one
type of process modelling system is
being put into practice.
Methods
The process was analysed by Puhos Board Oy and VTT. All
variables affecting the outcomes of particleboard were included in a database.
Data were collected and analysed with the Q-Opt program by Taipale Engineering.
Project information
Participants
Participants of the project, beside Puhos Board Oy, were the
Technical Research Centre Of Finland (VTT) and Taipale Engineering Ltd.
Project dates
The project started on 02.01.1997 and ended on 28.2.1999.
Project volume
Total budget of the project was about FIM 1 million.
Project manager
R&D Manager Esa Pesari
Teollisuustie 20 a, FIN-82430 PUHOS, Finland
Tel. +358 13 6823 247
Fax +358-13-6823 249
11.29 Using a neural network for boiler control
optimisation; a feasibility study
Goals
The goal of the project was to find out whether it is
possible to enhance boiler control by utilising artificial neural networks. The
reduction of NOx emissions from the Rauhalahti peat fuelled power plant was set
as a specific objective.
Results and impacts
An artificial neural network (NN) model was built in order
to determine how the NOx emissions are dependent on the manipulated variables
of the boiler.
The evaluation of the results brought up the following
facts.
- The NN model is consistent with the empirical
theoretical knowledge in a qualitative sense.
- Two NN models that are trained using different set of
measured data are not always consistent in a quantitative sense. This is
obviously due to the large variation in fuel properties, which is common in
peat and biofuel power plants.
We conclude that a neural network based combustion model is
feasible for NOx reduction in a peat fuelled or bio-fuelled boiler, provided
that it is adaptive. The model should adapt to new data in a rather short
period of time, for instance, in a few hours, or in a couple of days at the
latest. An informative system is probably more feasible than a closed loop
system.
Methods
Measured data from the boiler were acquired over several
months. The boiler was operated normally throughout this time, and no special
experiments were carried out.
A MLP network was created and trained using measured data.
The output variable of the network was the NOx content.
The input variables were certain manipulated
boiler variables (e.g. fuel feed rate, primary airflow and secondary airflow).
The dependencies between the NOx content and the manipulated
variables were determined by performing a sensitivity analysis with the model.
Project information
Participants
- Fortum Power and Heat Oy, formerly Imatran Voima Oy
- Taipale Engineering Oy
Project dates
Jan 1, 1995 to Dec 31, 1996
Project volume
FIM 150.000
Project manager
Martti Välisuo, M.Sc. (Tech)
Fortum Power and Heat Oy
Technology Centre
P.O. Box 20, FIN-00048 FORTUM, Finland
Publications
Välisuo, Kuoppa,
Taipale: Leijupolton optimointi neuroverkkomallin avulla. Automaatiopäivät
1995. (In Finnish.)

jukka.iivarinen@hut.fi
http://www.cis.hut.fi/neuronet/Tekes/11.shtml
Tuesday, 05-Dec-2000 12:56:31 EET
|